Labeling Tools for Machine Learning: Unlocking the Power of Data
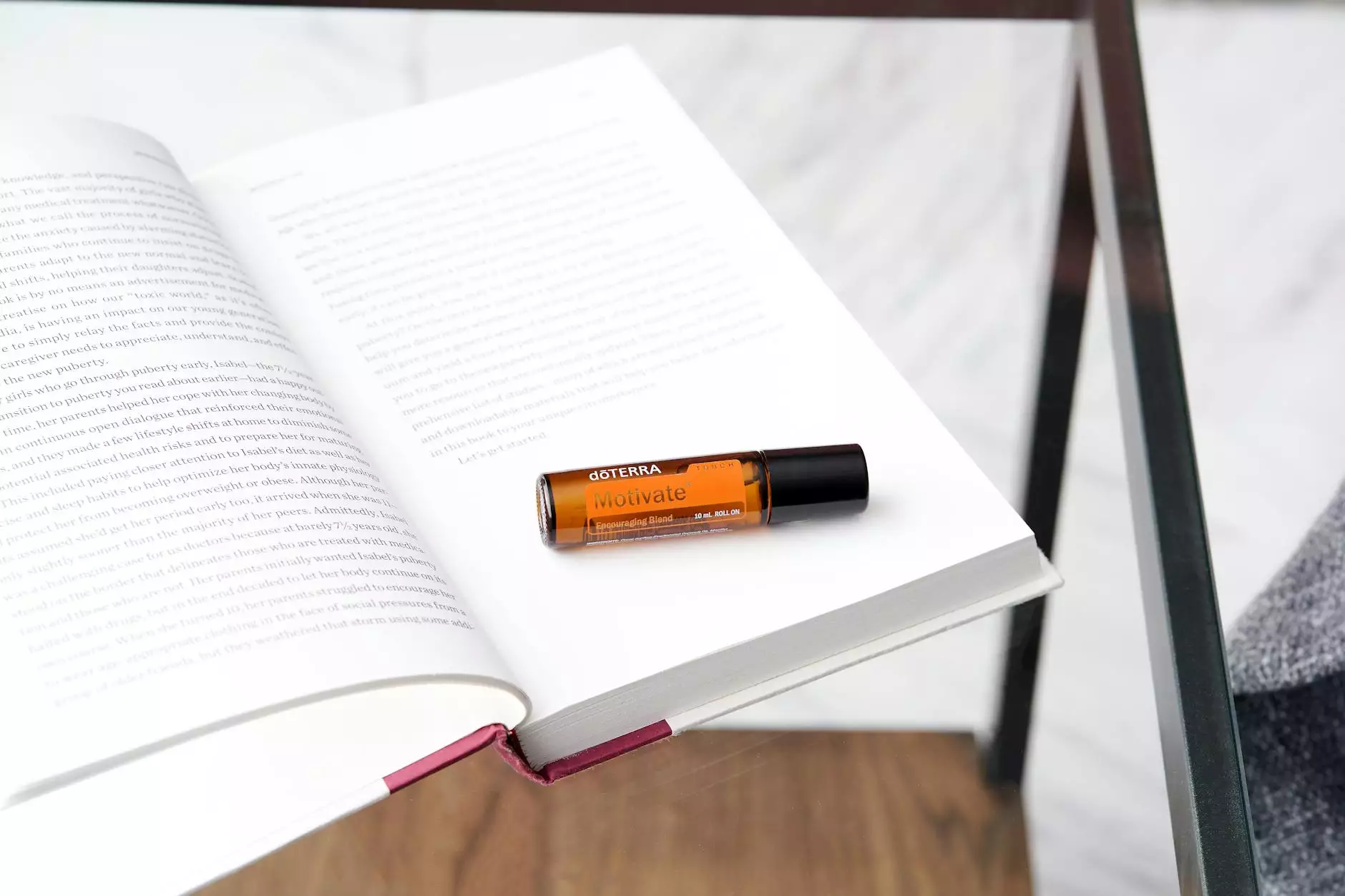
In the ever-evolving world of artificial intelligence (AI) and machine learning (ML), the data you use is crucial. One of the most important steps in any machine learning project is data annotation, which is essential for training accurate and reliable AI models. In this article, we'll explore various labeling tools for machine learning, their functionalities, and how they can enhance your ML projects. We aim to provide an informative guide that not only sheds light on the intricacies of data annotation but also highlights the best practices in selecting these tools.
What is Data Annotation?
Data annotation refers to the process of labeling data to make it understandable and usable for machine learning algorithms. Labeling involves tagging data points—such as images, texts, audio, and video—with relevant information that helps AI models learn and make decisions. The quality and accuracy of these labels directly influence the performance of the AI models.
The Importance of Data Annotation in Machine Learning
Data is the backbone of machine learning. Without properly annotated data, training models becomes challenging, if not impossible. Here’s why data annotation is vital:
- Model Accuracy: Well-labeled data leads to more accurate machine learning models, as they can better recognize patterns and make predictions.
- Algorithm Efficiency: Proper labels help in tuning algorithms for optimal performance, making your models more efficient.
- Scalability: As the volume of data grows, having a robust system of labeling tools allows for scalable data annotation processes.
- Compliance and Ethics: In today’s AI landscape, ensuring that data is labeled ethically and in compliance with regulations is paramount.
Types of Labeling Tools for Machine Learning
Labeling tools can be classified into different categories based on their functionality. Below are some common types:
1. Manual Labeling Tools
These tools require human intervention for labeling data. They are often used for complex tasks that require nuanced understanding, such as sentiment analysis in text or object detection in images. Examples include:
- Labelbox
- Amazon SageMaker Ground Truth
2. Automated Labeling Tools
Automated tools use algorithms to perform data labeling, significantly speeding up the process. However, they may require human oversight to ensure accuracy. Examples include:
- Snorkel
- SuperAnnotate
3. Integrated Annotation Platforms
These platforms combine various tools and workflows into a single interface, making it easier for teams to collaborate on labeling projects. Examples include:
- Keylabs.ai
- V7 Labs
Key Features to Look for in Labeling Tools
When selecting the right labeling tools for machine learning, there are several features you should consider:
1. User-Friendly Interface
A straightforward and intuitive user interface can significantly enhance productivity. Teams should be able to navigate the tool easily, regardless of their technical expertise.
2. Collaboration Capabilities
Effective collaboration features allow multiple team members to work together seamlessly, providing tools for communication and shared progress tracking.
3. Integration with ML Frameworks
Choosing tools that integrate well with popular machine learning frameworks (like TensorFlow, PyTorch, etc.) streamlines the workflow and saves time.
4. Quality Control Mechanisms
Tools should have built-in quality control processes to ensure the accuracy of labels. This could include review systems, revision logs, and metrics for checking label quality.
Best Practices for Data Annotation
To make the most of your labeling tools, follow these best practices:
1. Define Clear Guidelines
Establish clear labeling guidelines to ensure consistency. All team members should have a uniform understanding of how to annotate data.
2. Utilize Diverse Data Sets
When training your models, use diverse and representative data sets. This helps in reducing bias and improving model generalization.
3. Regular Feedback and Iteration
Feedback is vital. Regularly review the annotated data and offer constructive feedback to data annotators to enhance their labeling quality.
4. Stay Updated with Trends
The field of machine learning is dynamic. Stay informed about the latest trends in data annotation and AI to adopt newer, more efficient strategies.
A Case Study: Keylabs.ai's Impact on Data Annotation
Keylabs.ai stands out as a pivotal player in the realm of data annotation tools. With its robust data annotation platform, it provides a comprehensive solution that streamlines the process, enhances accuracy, and scales efficiently with the growing data needs of businesses.
The platform offers:
- Versatile Annotation Tools: Support for various data types such as images, texts, audio, and video.
- Collaborative Features: Teams can work in real-time, share insights, and review annotations collectively.
- Quality Assurance: Integrated quality control features help maintain high standards in labeling.
With its commitment to innovation, Keylabs.ai not only simplifies the data annotation process but also empowers organizations to leverage their data effectively, ultimately enhancing their AI capabilities.
Conclusion
Investing in effective labeling tools for machine learning is essential for any organization looking to harness the power of AI. By understanding the types of tools available, their features, and best practices in data annotation, businesses can make informed decisions that lead to superior model performance. As the landscape of machine learning continues to grow, utilizing platforms such as Keylabs.ai can provide a competitive edge, ensuring that your data is not just abundant but also of high quality.
In conclusion, the future of AI relies heavily on how well we annotate our data. Choose wisely, annotate accurately, and unlock the true potential of your machine learning projects.