Unlocking the Power of Video Annotation Tools for Machine Learning
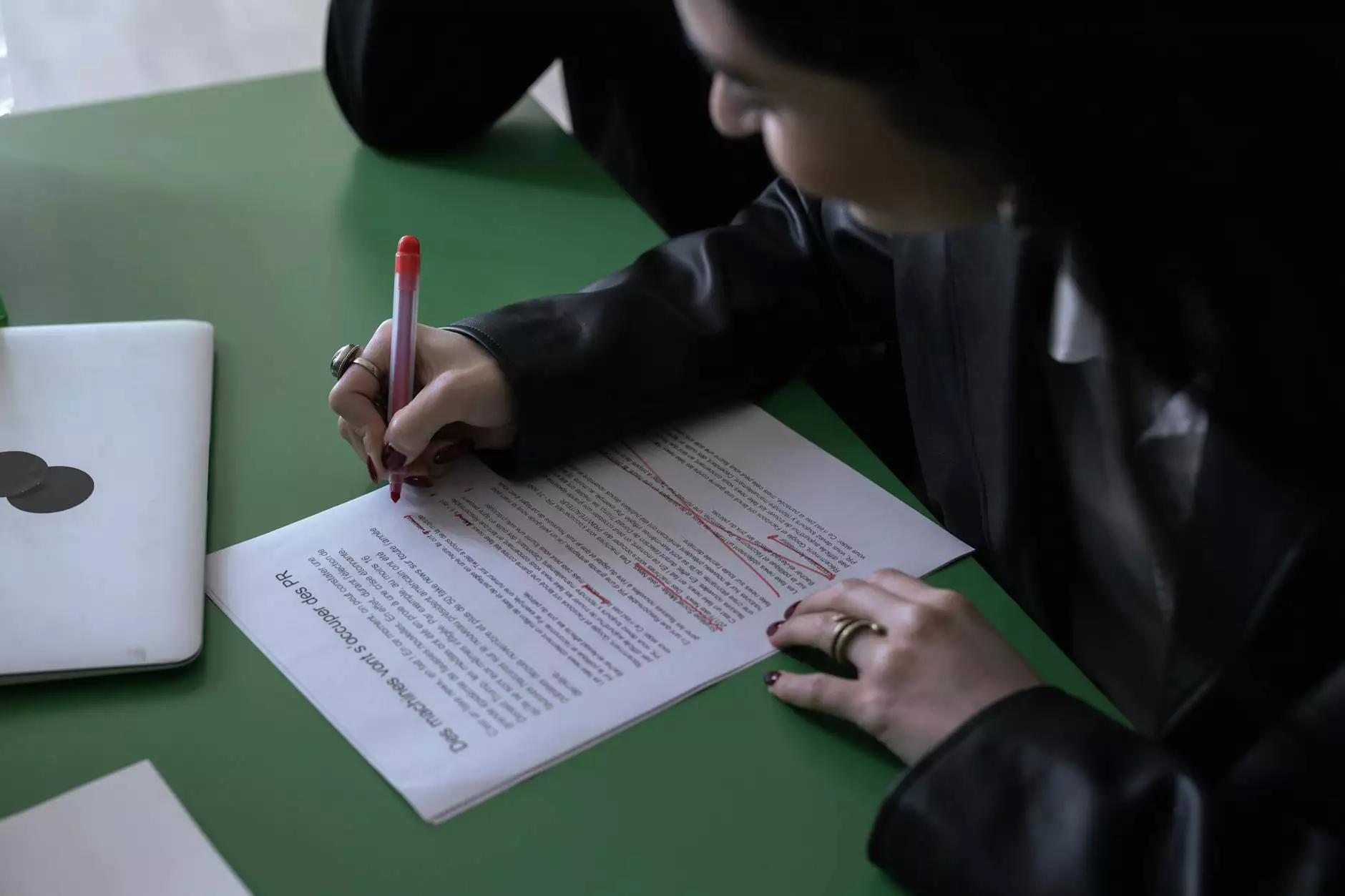
In today’s rapidly evolving tech landscape, the relationship between video annotation tools for machine learning and artificial intelligence (AI) is more crucial than ever. As businesses increasingly depend on machine learning to create intelligent systems, the need for efficient data labeling and annotation continues to grow. The precision of these tools plays a paramount role in the performance of machine learning models.
What is Video Annotation?
Video annotation refers to the process of labeling video content to create training datasets for machine learning algorithms. This involves identifying and tagging objects, actions, and other significant features within video frames. The importance of this process cannot be overstated, as accurately annotated video data is essential for improving the performance and accuracy of AI systems.
Why Utilize Video Annotation Tools?
Video annotation tools provide a comprehensive solution for various sectors looking to enhance their machine learning models. Here are several reasons why businesses should consider implementing these tools:
- Improved Data Quality: Effective annotation strategies ensure high-quality data, ultimately leading to better model outcomes.
- Time Efficiency: Automated annotation tools speed up the labeling process, allowing businesses to focus on analysis rather than manual data entry.
- Scalability: With the growing amount of video data being generated, scalable solutions allow for handling large datasets without compromising quality.
- Enhanced Collaboration: Most video annotation tools come with features that allow multiple team members to collaborate effectively, making it easier to manage projects.
The Applications of Video Annotation in Machine Learning
Video annotation tools are utilized across various industries, each with unique applications:
1. Autonomous Vehicles
In the automotive industry, video annotation is indispensable for developing self-driving car technology. Annotating video footage of real-world driving scenarios helps train models to recognize traffic signs, pedestrians, and potential obstacles, thus enhancing safety and reliability.
2. Surveillance Systems
Security systems leverage video annotation tools to enhance their monitoring capabilities. Annotated video footage can help in behavior analysis and anomaly detection, leading to improved response times and better overall security measures.
3. Sports Analysis
Sporting organizations utilize video annotation for performance analysis. Annotating gameplay footage enables teams to dissect player movements, strategies, and in-game decisions, helping to enhance performance through data-driven insights.
4. Healthcare
In healthcare, video annotation tools can be applied to medical imagery to train AI systems in diagnostic tasks. For instance, video footage of surgeries can be annotated to help in training robots for surgical assistance, thus improving surgical precision.
Types of Video Annotation Tools
There are several types of video annotation tools available in the market, each serving different needs:
- Manual Annotation Tools: Require a human annotator to label the video content frame by frame. Ideal for high-level precision tasks but can be time-consuming.
- Automated Annotation Tools: Use AI algorithms to recognize and label content automatically. While faster, they may compromise on accuracy, especially with complex scenes.
- Hybrid Tools: Combine both manual and automated processes, providing flexibility and efficiency.
Key Features to Look For
Choosing the right video annotation tool is essential for optimizing your machine learning initiatives. Here are key features to consider:
1. User-Friendly Interface
A clean, intuitive interface can significantly improve productivity. Look for tools that are easy to navigate, reducing the learning curve for new users.
2. Annotation types
Different projects require different annotation types. Ensure the tool supports various options such as bounding boxes, polygons, and points.
3. Export Options
Check if the tool offers flexible data export options, allowing you to easily integrate your annotated data with existing machine learning frameworks.
4. Video Handling Capability
The tool should support various video formats and have the capability to handle high-resolution videos without performance issues.
The Future of Video Annotation Tools
As machine learning progresses, the evolution of video annotation tools will be pivotal. With the continuous increase in video data generated globally, the demand for effective annotation solutions will only grow. Future advancements may focus on:
- AI-Powered Automation: Enhanced machine learning models capable of recognizing and annotating videos with minimal human involvement.
- Real-Time Annotation: Tools that can provide real-time annotation for live video feeds, especially beneficial in surveillance and security applications.
- Integration with Virtual Reality (VR): Expanding the use of annotation tools in VR environments for training simulations and immersive learning.
Conclusion
Video annotation tools for machine learning represent a vital component in the ongoing development of AI technologies. As the demand for annotated video data surges, businesses across various sectors are embracing these tools to improve accuracy, efficiency, and collaboration. By investing in robust video annotation solutions, organizations can better position themselves in the competitive landscape of machine learning.
Enhancing your machine learning models with high-quality video data is crucial for achieving better outcomes. With the right tools and strategies, the benefits of video annotation can unlock new frontiers of possibility across industries, driving innovation and growth.
video annotation tool for machine learning