The Ultimate Guide to Image Labeling Tools for Object Detection
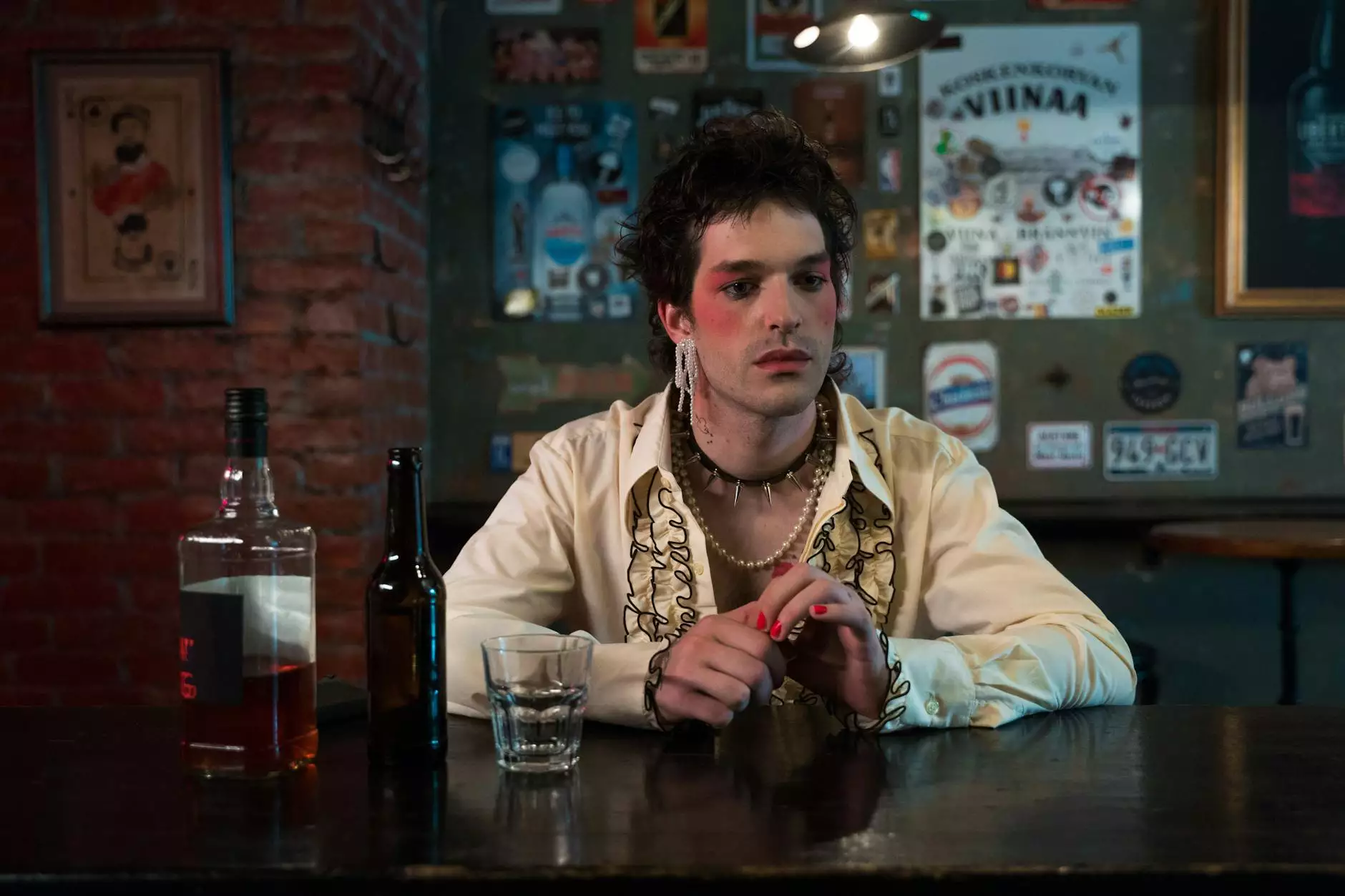
The world of artificial intelligence (AI) and machine learning (ML) is rapidly evolving, with object detection rising to the forefront of these advancements. The foundation of successful object detection algorithms relies heavily on the quality of the training data provided. This makes the role of an image labeling tool for object detection indispensable for businesses and researchers alike.
Understanding the Importance of Image Labeling
In any AI project focusing on visual data, the first critical step is labeling the images correctly. This process involves annotating parts of an image to identify objects, which helps in training ML models. High-quality image labeling tools facilitate accurate annotation, which in turn enhances the model's ability to recognize and classify objects accurately.
What is an Image Labeling Tool?
An image labeling tool is a software application designed to assist in the annotation of images. These tools vary in functionality, providing features that cater to both manual and automated labeling processes. Through intuitive interfaces and advanced technologies, image labeling tools streamline the data annotation workflow, enabling users to focus on achieving high-quality outputs efficiently.
Benefits of Using Image Labeling Tools
- Increased Efficiency: Manual labeling can be a cumbersome process. Image labeling tools enable quicker annotation through various features such as batch processing and smart suggestions.
- Enhanced Accuracy: Tools often incorporate AI assistance that reduces human error, ensuring more accurate labels over time.
- Collaboration Features: Many platforms provide options for multiple users to collaborate, making it easier for teams to manage large datasets.
- Customization: Users can customize labeling tools to fit their specific project needs, such as defining unique classes or shapes.
- Scalability: As data needs grow, these tools can scale to accommodate larger projects while maintaining performance.
Key Features of an Effective Image Labeling Tool for Object Detection
Choosing the right image labeling tool involves understanding which features will best meet your needs. Here are some essential features to consider:
1. User-Friendly Interface
A clean and intuitive user interface (UI) is paramount. Users should be able to navigate the tool effortlessly without extensive training.
2. Support for Multiple Annotation Types
Object detection tasks often require various types of annotations, including bounding boxes, polygons, and keypoints. A versatile tool should support all these types.
3. Integration with Machine Learning Frameworks
The ability to export annotated data in formats compatible with popular ML frameworks (like TensorFlow, PyTorch, etc.) enhances a tool's usability.
4. Automated Labeling Features
Some advanced tools offer AI-powered auto-labeling that suggests labels based on previously annotated examples, significantly speeding up the process.
5. Quality Assurance Capabilities
Features that allow for review and validation of annotations help maintain low error rates, which is crucial for training robust models.
Exploring Keylabs.ai: A Premier Data Annotation Platform
One of the leading platforms in the market for image labeling is Keylabs.ai. This innovative platform excels in providing comprehensive solutions for data annotation, specifically tailored for object detection.
Why Choose Keylabs.ai?
Keylabs.ai stands out due to its commitment to quality and efficiency in data annotation. Here’s why it’s the go-to platform for many businesses:
- Expert Annotation Team: Keylabs.ai employs skilled annotators who ensure that every image is labeled with precision.
- Advanced Technology: The platform integrates cutting-edge machine learning technologies to enhance annotation speed and accuracy.
- Custom Solutions: Businesses can tailor the annotation process to meet specific project requirements, ensuring the best fit.
- Robust Support: Keylabs.ai provides exceptional customer support, assisting users at every step of their annotation journey.
The Workflow of Image Labeling for Object Detection
Understanding the typical workflow for image labeling can help stakeholders ensure they follow best practices:
Step 1: Dataset Preparation
Start by collating all the images that need to be labeled. Ensure the dataset reflects the diversity of objects and conditions found in real-world scenarios.
Step 2: Choosing the Right Tool
Select a robust image labeling tool, such as those offered by Keylabs.ai, which aligns with your project’s requirements.
Step 3: Annotation Process
Begin annotating the dataset using the selected tool. Utilize features like bounding boxes or segmentation masks to accurately represent the boundaries of objects within images.
Step 4: Review and Quality Assurance
Implement a stringent review process where annotated images are double-checked for accuracy. This step dramatically impacts the quality of the final model.
Step 5: Exporting Data
Once annotations are complete, export the data in a compatible format to be used in training machine learning models.
Challenges in Image Labeling and How to Overcome Them
While image labeling plays a critical role in object detection, it comes with its own set of challenges. Here are some challenges and potential solutions:
1. Subjectivity in Labeling
Labelers may interpret images differently, which can lead to inconsistencies. Establishing a clear set of guidelines and examples can help unify the labeling process.
2. Time Consumption
Manually annotating large datasets can be time-consuming. Utilizing an image labeling tool with AI-assisted features can streamline the process considerably.
3. Handling Diverse Data
Diverse data can introduce complexity in labeling. A comprehensive tool should provide features for annotating varying image conditions and perspectives.
Future Trends in Image Labeling and Object Detection
As technology continues to evolve, so do image labeling methodologies. Here are some emerging trends:
1. AI-Driven Annotation
With advancements in AI, automated image labeling capabilities are becoming more sophisticated, allowing for quicker and more reliable annotations with less human intervention.
2. Real-Time Labeling
As applications demand faster processing, real-time image labeling is becoming more feasible, especially in dynamic environments.
3. Collaborative Annotation Platforms
Enhanced collaborative features will simplify teamwork, making it easier for different stakeholders to work on the same projects seamlessly.
4. Enhanced Integration Capabilities
Future tools will likely offer greater integration with various data lakes and ML platforms, creating a more unified workflow for data scientists and engineers.
Conclusion
In today’s data-driven world, the significance of an effective image labeling tool for object detection cannot be overstated. With platforms like Keylabs.ai, organizations can streamline their data annotation processes, ensuring that their machine learning models are trained on accurate and high-quality data. By harnessing the power of advanced image labeling software, businesses can stay ahead of the curve and leverage the full potential of AI technology. Start your journey towards enhanced object detection today with the right tools and strategies!