Unlocking the Power of Image Annotation Tools in Machine Learning
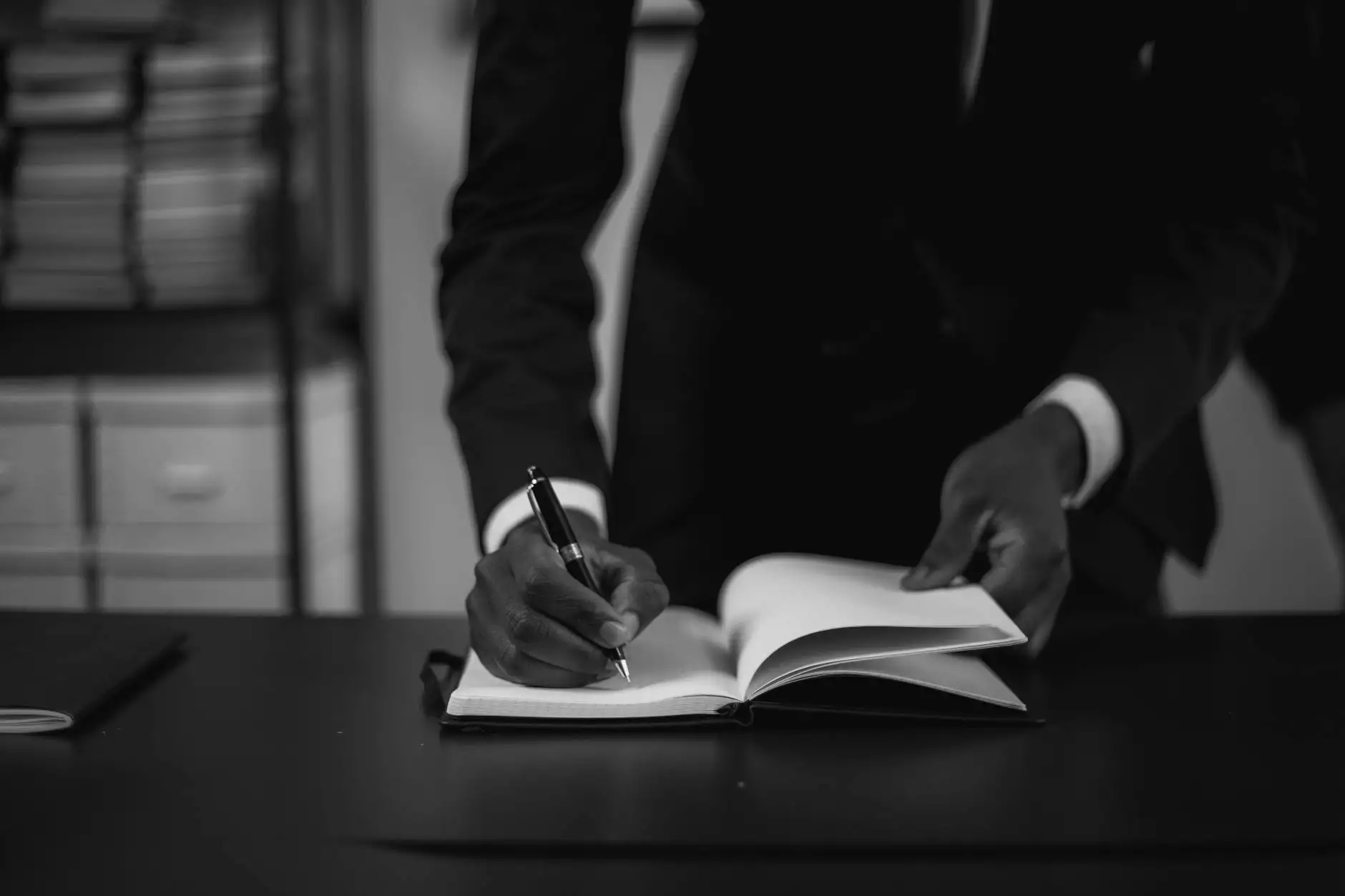
Machine learning has revolutionized various sectors, notably through the advent of sophisticated image annotation tools. These tools are critical in enhancing the quality and accuracy of datasets, enabling artificial intelligence systems to learn effectively. In this article, we will delve deep into the significance of image annotation tools in machine learning, focusing on their impact, methods, and how businesses like Keymakr leverage these technologies to stay ahead in the market.
Understanding Image Annotation
At its core, image annotation refers to the process of labeling images for the purpose of training machine learning models. This labeled data is crucial because machine learning algorithms learn from the examples presented to them. The more accurately an image is annotated, the better the model can predict or categorize new, unseen data.
Types of Image Annotations
Image annotation can take various forms depending on the requirements of the machine learning task at hand. Common types include:
- Bounding Boxes: Used for detecting objects in images, creating rectangular boxes around the objects of interest.
- Semantic Segmentation: Every pixel in the image is labeled with a class, creating a precise mask that defines the objects within the image.
- Keypoint Annotation: Used to mark specific points within an image, typically for applications like pose estimation.
- Polygonal Annotation: Offers a more detailed outline by creating complex shapes around objects that cannot be easily encompassed by a rectangle.
- Image Classification: Labels an entire image to indicate its overall category or class.
The Role of Image Annotation Tools in Machine Learning
The proficiency of an image annotation tool significantly impacts the quality of the training data. High-quality annotations lead to superior model performance. Here’s how these tools are pivotal in the development of machine learning applications:
1. Enhanced Data Quality
Quality is paramount in machine learning. With the right annotation tools, organizations ensure that the data fed into machine learning algorithms is accurate and relevant. This enhancement is vital for tasks like object detection, where precision in labeling can determine the success of the entire model.
2. Efficiency in Annotation Processes
Manual image annotation can be labor-intensive and time-consuming. Advanced image annotation tools automate various aspects of the annotation process, significantly reducing the time needed to prepare datasets. This efficiency allows businesses to dedicate more resources to other critical areas of software development.
3. Scalability
As machine learning needs expand, so do the datasets required. Annotation tools provide scalability, allowing businesses to handle vast amounts of imagery without a proportional increase in workload. This adaptability is essential for companies developing complex models requiring extensive and diverse datasets.
4. Consistency and Accuracy
Manual errors are commonplace in the annotation process. However, powerful image annotation tools utilize algorithms and machine learning techniques to maintain consistency and accuracy across the board. This feature is especially crucial when producing datasets that require high reliability for critical applications such as healthcare, autonomous driving, and security surveillance.
Choosing the Right Image Annotation Tool
When selecting an image annotation tool for machine learning, businesses must consider several factors to ensure they meet their unique needs:
1. User Interface and Usability
A user-friendly interface allows team members to learn the tool quickly and use it effectively, reducing training time. Good annotation tools should enable intuitive navigation and simple workflows.
2. Types of Annotations Supported
Depending on the application, organizations may require specific annotation types. Ensure that the chosen tool supports all necessary formats, including bounding boxes, segmentation, and polyline annotations.
3. Integration Capabilities
The ability of the annotation tool to integrate seamlessly with existing machine learning frameworks and workflows is crucial for operational efficiency. Tools that can connect with libraries like TensorFlow, PyTorch, or data management systems add significant value.
4. Collaboration Features
In many scenarios, teams composed of multiple individuals will work on projects. Tools offering collaboration features facilitate smooth communication and information sharing between team members, thereby improving overall productivity.
5. Advanced Features
Consider tools that offer more than basic functionalities, such as AI-assisted annotations, automated labeling, or pre-trained models that can speed up the annotation process. These features contribute significantly to reducing time and effort while enhancing quality.
Impact of Image Annotation on Software Development
The influence of image annotation tools extends beyond just machine learning; they play a crucial role in the overall software development lifecycle. Here are some ways in which they impact software development:
1. Rapid Prototyping
Image annotation tools enable swift iteration and development of prototypes. This rapid development cycle ensures that software features can be tested and reinforced with more robust datasets, leading to better performance.
2. Improved Collaboration Between Data Scientists and Developers
A well-annotated dataset bridges the gap between data scientists and software developers. By providing clean, accurate data, developers can create machine learning models that are ready for integration, leading to smoother workflows and better outcomes.
3. Feedback Loops
Quality annotations provide a foundation for establishing feedback loops, allowing data scientists to refine their models continuously. With image annotations serving as a reliable input, models can adapt and learn much faster.
Advantages of Using Keymakr for Image Annotation
Businesses seeking to capitalize on machine learning must select reliable partners in their quest for superior data collection and annotation. Keymakr stands as a leading provider of robust image annotation tools designed for optimal integration and efficiency.
- Expertise: With years of experience in the industry, Keymakr understands the nuances involved in image annotation for machine learning applications.
- User-Friendly Platform: Keymakr’s tools boast a simple interface enabling efficient workflows, making the annotation process quick and manageable.
- Quality Assurance: Consistency and accuracy are at the forefront of Keymakr’s ethos, ensuring every project meets high standards.
- Customization: Keymakr offers customization options to meet unique project needs, allowing for tailored solutions that enhance data quality.
- Collaboration Tools: The platform encourages team synergy, with features designed for easy sharing and feedback.
Conclusion
In the rapidly evolving world of technology, the significance of image annotation tools in machine learning cannot be overstated. These tools are not merely supportive; they are foundational to creating accurate, reliable datasets needed for training effective models. As businesses like Keymakr continue to innovate in this space, the potential for advancements in machine learning applications becomes boundless.
By investing in high-quality image annotation tools, companies can enhance their software development capabilities and ensure that they remain competitive in an increasingly data-driven landscape. The future of machine learning relies on how well we can harness the power of expertly annotated data—making the right choice in annotation tools a crucial step toward success.
image annotation tool machine learning